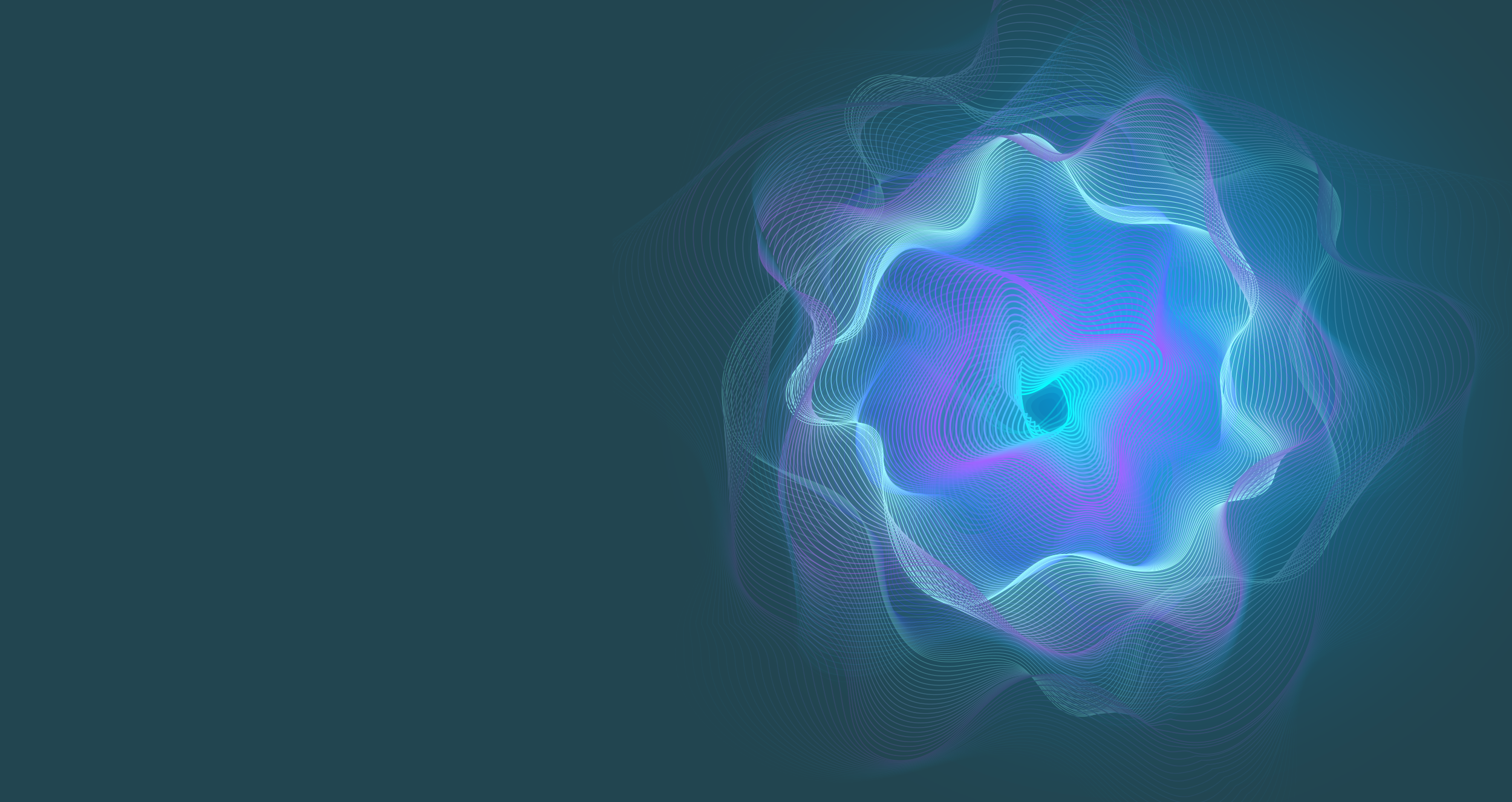
BY OPENING UP THE BLACK BOX OF AI, WE CREATE FULL EXPLAINABILITY
AND TRANSPARENCY
Black box AI is any artificial intelligence system whose inputs and operations are not visible to the user or another interested party. A black box, in a general sense, is an impenetrable system.
Deep learning modeling is typically conducted through black box development: The algorithm takes millions of data points as inputs and correlates specific data features to produce an output. That process is largely self-directed and is generally difficult for data scientists, programmers and users to interpret.
When the workings of software used for important operations and processes within an organization cannot easily be viewed or understood, errors can go unnoticed until they cause problems so large that it becomes necessary to investigate and the damage caused may be expensive or even impossible to repair.
In language, for example — one of the most obvious sources of bias is when algorithms learn from written text. They pick up some associations between words that appear together more often. They learn, for example, that “man is to computer programmer as woman is to homemaker.” When this algorithm is tasked to find the right résumé for a programming job, it will be more likely to pick male applicants than females.
AI bias, for example, can be introduced to algorithms as a reflection of conscious or unconscious prejudices on the part of the developers, or they can creep in through undetected errors. In any case, the results of a biased algorithm will be skewed, potentially in a way that is offensive to people who are affected. Bias in an algorithm may come from training data when details about the dataset are unrecognized. In one situation, AI used in a recruitment application relied upon historical data to make selections for IT professionals. However, because most IT staff historically were male, the algorithm displayed a bias toward male applicants.
If such a situation arises from black box AI, it may persist long enough for the organization to incur damage to its reputation and, potentially, legal actions for discrimination. Similar issues could occur with bias against other groups as well, with the same effects. To prevent such harms, it's important for AI developers to build transparency into their algorithms and for organizations to commit to accountability for their effects.
“Black box” = There’s no way to determine how the algorithm came to your decision.
AI YOU CAN TRUST #NOBLACKBOX
Artificial Intelligence has been around for 50+ years with a massive amount of progress recently by researchers, companies and institutions. We've seen major milestones and tremendous change in computational linguistics, natural language processing, semantic modelling and of course machine learning. The rise of deep learning has accelerated the dawn of AI and we anticipate an even more rapid acceleration of AI achievements in the next decade. We’ve invested and combined these techniques to deliver a unique approach to using natural language understanding to benefit businesses.
Central to our company values at Nalantis is a strong belief in AI transparency… AI you can Trust. Putting trust at the center will help build better AI frameworks avoiding ill performance or biased algorithms.
Implementing transparency and explainable AI helps Enterprise and government understand how their data is being used and how decisions are made. What makes this challenging is the growing complexity of machine learning and the popularity of deep-learning neural networks, which can behave like black boxes with no explanation of how their results were computed.
The NoBlackbox approach at Nalantis (Semantic ConceptNet Modeling) enables the developer community to tap into APIs for semantic representation of language and unstructured text and creates full explainability and transparency.
BY OPENING UP THE BLACK BOX OF AI, WE CREATE FULL EXPLAINABILITY AND TRANSPARENCY.
The characteristics analytics executives value most when developing AI’s
How important are the following characteristics for ensuring business success when developing an AI product?
5 = Most important
1 = Least important
Source: Building AI-Driven Enterprises in a Disrupted Environment — 2020 FICO & Corinium
The NoBlackbox approach, as opposed to black box AI, provides transparency for the part of the artificial intelligence process where algorithms interpret data.
Source: Ditto
This means two main business problems are solved:
Accountability – we know how an automated decision is reached and can trace the path of reasoning if needed.
Auditability – we can review processes, test, and refine them more accurately, and predict and prevent future failures or gaps.
Ethical AI black box problems complicate user trust in the decision making of algorithms. As AI looks to the future, experts urge developers to take a glass box approach.
Subscribe to our newsletter.
Sign up with your email address to receive news and updates.